Note
Go to the end to download the full example code
Release Highlights for tttrlib 0.23¶
We are pleased to announce the release of tttrlib 0.20, which comes with many bug fixes and new features! We detail below a few of the major features of this release. For an exhaustive list of all the changes, please refer to the release notes.
To install the latest version with conda:
conda install -c tpeulen tttrlib
Test for plotting¶
The inspection.permutation_importance
can be used to get an
estimate of the importance of each feature, for any fitted estimator:
import numpy as np
import matplotlib.pyplot as plt
fig, ax = plt.subplots()
x = np.linspace(0, 5)
ax.plot(x, np.sin(x))
ax.set_title("Permutation Importance of each feature")
ax.set_ylabel("Features")
fig.tight_layout()
plt.show()
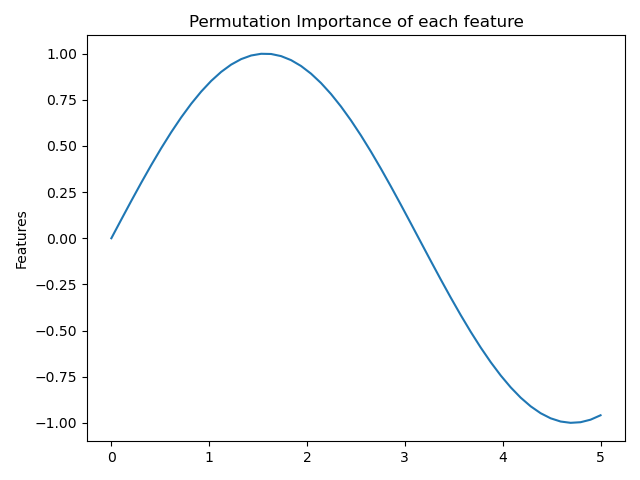
Improved PTU header support¶
The ensemble.HistGradientBoostingClassifier
and ensemble.HistGradientBoostingRegressor
now have native
support for missing values (NaNs). This means that there is no need for
imputing data when training or predicting.
print("Better Header support")
Better Header support
Writing of TTTR data¶
Most estimators based on nearest neighbors graphs now accept precomputed
sparse graphs as input, to reuse the same graph for multiple estimator fits.
To use this feature in a pipeline, one can use the memory
parameter, along
with one of the two new transformers,
neighbors.KNeighborsTransformer
and
neighbors.RadiusNeighborsTransformer
. The precomputation
can also be performed by custom estimators to use alternative
implementations, such as approximate nearest neighbors methods.
See more details in the User Guide.
from tempfile import TemporaryDirectory
import tttrlib
# with TemporaryDirectory(prefix="tttrlib_temp_") as tmpdir:
# estimator = make_pipeline(
# KNeighborsTransformer(n_neighbors=10, mode='distance'),
# Isomap(n_neighbors=10, metric='precomputed'),
# memory=tmpdir)
# estimator.fit(X)
#
# # We can decrease the number of neighbors and the graph will not be
# # recomputed.
# estimator.set_params(isomap__n_neighbors=5)
# estimator.fit(X)
Total running time of the script: (0 minutes 0.231 seconds)