Note
Click here to download the full example code
Accessible volume decorator¶
IMP particles can be decorated with accessible volume (AV) decorators to compute the sterically allowed volume of a label around its attachment site, i.e., the decorated IMP particle.
The AV decorator uses the Dijkstra’s algorithm to compute
all path from the attachment site to a set of grid points
around the attachment site. The AV decorator uses the
IMP.bff.PathMap
class for computing the AV. The occupied
volume of leaves in a molecular hierarchy serve as obstacles
in the path search.
import pathlib
import numpy as np
import pylab as plt
import IMP
import IMP.core
import IMP.atom
import IMP.em
import IMP.bff
Setup Model¶
m = IMP.Model()
pdb_fn = pathlib.Path(IMP.bff.get_example_path('structure')) / "T4L/3GUN.pdb"
hier = IMP.atom.read_pdb(str(pdb_fn), m)
Decorate particle¶
Any particle can be decorated by AVs. The location of the AV particle is changed by AV calculation to the mean position of the AV. Thus, better create a new particle that will be decorated with an AV.
av_p = IMP.Particle(m)
An AV has a source, i.e., the labeling site and a set of parameters that determine the shape of the AV. We select
residue_index = 132
atom_name = "CB"
sel = IMP.atom.Selection(hier)
sel.set_atom_type(IMP.atom.AtomType(atom_name))
sel.set_residue_index(residue_index)
source = sel.get_selected_particles()[0]
av_parameter = {
"linker_length": 20.0,
"radii": (3.5, 0.0, 0.0),
"linker_width": 0.5,
"allowed_sphere_radius": 2.0,
"contact_volume_thickness": 0.0,
"contact_volume_trapped_fraction": -1,
"simulation_grid_resolution": 0.5
}
IMP.bff.AV.do_setup_particle(m, av_p, source, **av_parameter)
av1 = IMP.bff.AV(m, av_p)
The coordinates of an AV are the mean AV the density map. Thus, the position of the AV changes when the AV is resampled.
av_xyz = IMP.core.XYZ(av1)
# av_mp == (0,0,0)
av1.resample() # Updates the AV
# av_xyz == (-1.46132, -25.366, -6.04022)
Access AV features¶
AV decorated particles use PathMaps to sample the accessible volume. Thus, features of the AV are accesses trough PathMap. The features can be written to density maps (see: PathMapTile). PathMaps derive from IMP EM density maps and sampled obstacles can be written to density files using standard IMP methods.
av_map = av1.get_map()
bounds = 0.01, 20
# write to map
#IMP.em.write_map(av_map, "OBSTACLES.mrc")
Features of a IMP.bff.PathMap are identified by the following constants
pm_features = [
IMP.bff.PM_TILE_PENALTY, # Penality of visiting a tile
IMP.bff.PM_TILE_COST, # Cost of a path to the tile
IMP.bff.PM_TILE_DENSITY, # Density of tile
IMP.bff.PM_TILE_COST_DENSITY, # Cost * Density of tile
IMP.bff.PM_TILE_PATH_LENGTH, # Path length to tile (cost * grid spacing)
IMP.bff.PM_TILE_PATH_LENGTH_DENSITY, # Path length to tile * density
IMP.bff.PM_TILE_FEATURE, # Additional feature of tile (accessed by name)
IMP.bff.PM_TILE_ACCESSIBLE_DENSITY, # Density of tiles with path length in bounds
IMP.bff.PM_TILE_ACCESSIBLE_FEATURE # Feature of tile with path length in bounds
]
These features can be written to density maps
# IMP.bff.write_path_map(
# av_map, "BFF_TILE_PENALTY.mrc",
# IMP.bff.PM_TILE_PENALTY,
# (0, 1)
# )
# PM_TILE_PATH_LENGTH_WEIGHT : filter by path length and write tile weight
# IMP.bff.write_path_map(
# av_map, "PM_TILE_PATH_LENGTH.mrc",
# IMP.bff.PM_TILE_PATH_LENGTH,
# bounds
# )
# IMP.bff.write_path_map(
# av_map, "PM_TILE_PATH_LENGTH_DENSITY_132.mrc",
# IMP.bff.PM_TILE_PATH_LENGTH_DENSITY,
# bounds
# )
# IMP.bff.write_path_map(
# av_map, "PM_TILE_ACCESSIBLE_DENSITY.mrc",
# IMP.bff.PM_TILE_ACCESSIBLE_DENSITY,
# bounds
# )
Measure AV/AV-distance¶
residue_index = 55
sel = IMP.atom.Selection(hier)
sel.set_atom_type(IMP.atom.AtomType("CB"))
sel.set_residue_index(residue_index)
p = sel.get_selected_particles()[0]
av_p2 = IMP.Particle(m)
IMP.bff.AV.do_setup_particle(m, av_p2, p, **av_parameter)
av2 = IMP.bff.AV(av_p2)
v = IMP.bff.av_distance(av1, av2)
print(v)
55.642224549950214
Distance types¶
distance_types = [
IMP.bff.DYE_PAIR_DISTANCE_E, # Mean FRET averaged distance R_E
IMP.bff.DYE_PAIR_DISTANCE_MEAN, # Mean distance <R_DA>
IMP.bff.DYE_PAIR_DISTANCE_MP, # Distance between AV mean positions
IMP.bff.DYE_PAIR_EFFICIENCY, # Mean FRET efficiency
IMP.bff.DYE_PAIR_DISTANCE_DISTRIBUTION, # (reserved for Distance distributions)
IMP.bff.DYE_PAIR_XYZ_DISTANCE # Distance between XYZ of dye particles
]
forster_radius = 52.0 # Optional (default 52.0)
n_samples = 20000 # Optional (default 10000)
fret_efficiency = IMP.bff.av_distance(
av1, av2,
forster_radius=forster_radius,
distance_type=IMP.bff.DYE_PAIR_EFFICIENCY,
n_samples=n_samples
)
distance_fret = IMP.bff.av_distance(
av1, av2,
forster_radius,
IMP.bff.DYE_PAIR_DISTANCE_E,
n_samples
)
mean_inter_dye_distance = IMP.bff.av_distance(av1, av2, distance_type=IMP.bff.DYE_PAIR_DISTANCE_MEAN)
print("Mean FRET efficiency : {:.2f}".format(fret_efficiency))
print("Distance FRET : {:.1f}".format(distance_fret))
print("Mean inter-dye distance: {:.1f}".format(mean_inter_dye_distance))
print("Distance mean position : {:.1f}".format(IMP.bff.av_distance(av1, av2, IMP.bff.DYE_PAIR_DISTANCE_MP)))
Mean FRET efficiency : 0.43
Distance FRET : 54.5
Mean inter-dye distance: 55.4
Distance mean position : 55.4
Distance distribution between two AVs
rda_start, rda_stop, n_bins = 0, 100, 128
n_samples = 10000
rda = np.linspace(rda_start, rda_stop, n_bins)
p_rda = IMP.bff.av_distance_distribution(av1, av2, rda, n_samples=n_samples)
plt.plot(rda, p_rda)
plt.show()
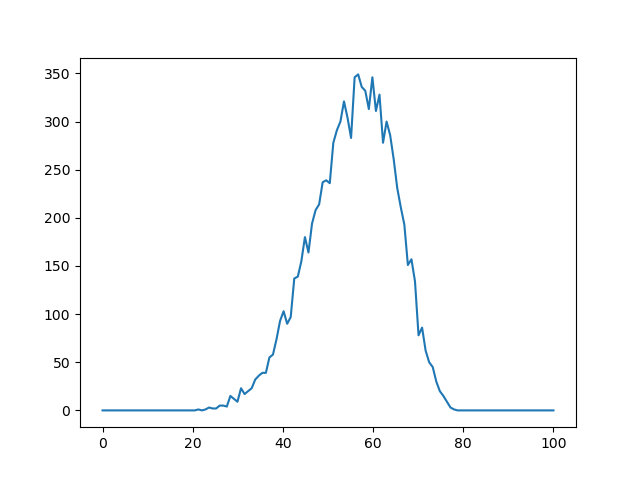
Total running time of the script: ( 0 minutes 2.696 seconds)