Note
Go to the end to download the full example code
Release Highlights for IMP.bff 0.12.0¶
We are pleased to announce the release of IMP.bff 0.12, which comes with many bug fixes and new features! We detail below a few of the major features of this release. For an exhaustive list of all the changes, please refer to the release notes.
To install the latest version with conda:
conda install -c tpeulen imp.bff
import numpy as np
import pylab as plt
import pathlib
import IMP
import IMP.core
import IMP.atom
import IMP.bff
import IMP.em
Path searches¶
The bff.PathMap
now supports A* and Djikstra path
searches to find. This means that optimal path between points
(e.g., for the surface accessible distances) for between
two points (A*) or multiple points (Djikstra)
can be searched.
m = IMP.Model()
pdb_fn = pathlib.Path(IMP.bff.get_example_path('structure')) / "T4L/3GUN.pdb"
hier = IMP.atom.read_pdb(str(pdb_fn), m)
sel = IMP.atom.Selection(hier)
sel.set_chain_id('A')
sel.set_residue_index(132)
sel.set_atom_type(IMP.atom.AtomType('CB'))
sel_particles = sel.get_selected_particles()
labeled_p = IMP.core.XYZ(sel_particles[0])
path_origin = labeled_p.get_coordinates()
path_map_header_parameter = {
"max_path_length": 20.0,
"grid_spacing": 1.0,
"neighbor_radius": 2,
"obstacle_threshold": 1e-5
}
path_map_header = IMP.bff.PathMapHeader(**path_map_header_parameter)
path_map_header.set_path_origin(path_origin)
path_map = IMP.bff.PathMap(path_map_header)
ps = [a.get_particle() for a in IMP.atom.get_leaves(hier)]
path_map.set_particles(ps)
path_map.sample_obstacles(extra_radius=0.0)
# The origin is located on an atom and hence blocked. Thus, there cannot be
# a path to the origin. Hence, unblock sphere around the origin.
path_map.fill_sphere(path_origin, radius=3.0, value=0.0, inverse=False)
path_map.update_tiles()
start_idx = path_map.get_voxel_by_location(path_origin)
path_map.find_path_dijkstra(start_idx, -1) # if the end_idx
t = path_map.get_tiles()
end_idx_1 = 829
path_1 = t[end_idx_1].backtrack_to_path()
print(path_1)
end_idx_2 = 229
path_2 = t[end_idx_2].backtrack_to_path()
print(path_2)
(32820, 31180, 29540, 27900, 24700, 21500, 18300, 16701, 15102, 13503, 10303, 8704, 7105, 5506, 3947, 2388, 829)
(32820, 31180, 29540, 27901, 26301, 24662, 23023, 21383, 19743, 18103, 16463, 13263, 11624, 9985, 8346, 6707, 5068, 3429, 229)
The most important attributes of tiles are the penalty and the cost of visiting a tile (from the starting point). Additionally, a tile has a density, e.g., used to implement weighted accessible volumes and a set of other (user-defined) features that are stored in a dictionary.
bounds = 0.0, 30
BFF_TILE_PENALTY = path_map.get_tile_values(IMP.bff.PM_TILE_PENALTY, (0, 1))
PM_TILE_COST = path_map.get_tile_values(IMP.bff.PM_TILE_COST, bounds)
PM_TILE_COST_DENSITY = path_map.get_tile_values(IMP.bff.PM_TILE_COST_DENSITY, bounds)
plt.imshow(PM_TILE_COST_DENSITY[16])
plt.show()
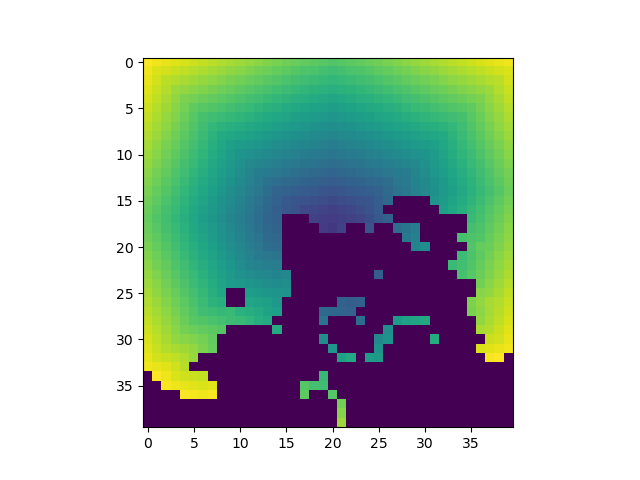
These features are returned as three dimensional arrays.
fig, axs = plt.subplots(1, 3, sharex=True)
axs[0].imshow(BFF_TILE_PENALTY[16])
axs[1].imshow(PM_TILE_COST[16])
axs[2].imshow(PM_TILE_COST_DENSITY[16])
plt.show()
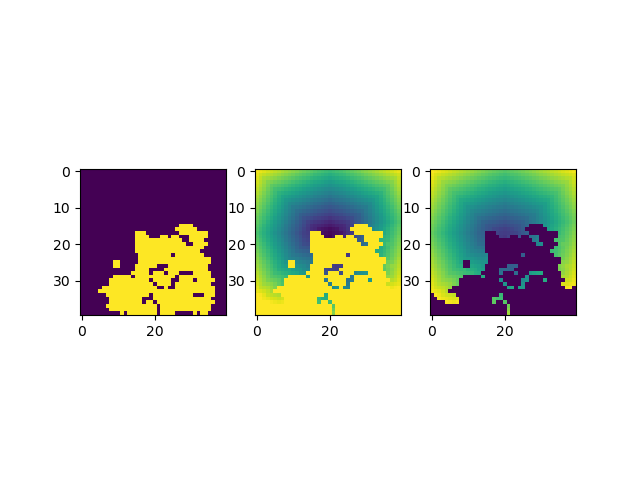
Total running time of the script: (0 minutes 0.188 seconds)